The world of forecasting today is filled with machine-related buzzwords like AI, predictive analytics and machine learning. Will all of forecasting be done by machines a few years from now? Do humans still have any competitive advantage over software? In the realm of new product forecasting – the human wins.
Human trumps machine forecasting in the following areas:
- Measuring promo impact
- Gauging life cycle trends
- Disentangling overlapping marketing tactics
- Incorporating qualitative analysis
Machines have great potential to improve forecasting processes. Automating forecasts for existing products that have a lot of data can free human time to focus on new product forecasting. However, engaging software in forecasting all products, both old and new, can pose serious risks for forecast accuracy.
How Much Value Does AI Really Add?
Hollywood has been questioning whether the machines will take over the world for almost half a century so it must be true. How will it start? Machines flipping burgers, executing court rulings or maybe doing demand planning? Demand planners are naturally intrigued about the latter. Industry trend-setters prefer to talk about AI fueled by machine learning algorithms over Excel books fueled by ridiculous amounts of coffee. Buzzwords sell, but do they really add value?
To answer that question we need to ask what AI and machine learning are. In the context of forecasting, these disciplines are essentially a series of algorithms that create baseline models and measure promotional impacts. The ‘machine learning’ component is a fancy term for the trivial process of feeding the algorithm with more data. These tools are very useful for forecasting products with lots of history and homogeneous promotions. These types of products are usually the easiest to forecast. Forecast error for products with lots of history is typically low, regardless of the level of automation. The tools save a lot of time and brain cells from doing tedious repetitive tasks, but they hardly improve forecast accuracy.
Can Algorithms Work With Little To No Sales Data?
But what about new products that have no or little sales data? Ironically these are also the ones that are subject to all kinds of marketing extremities. Can any machine algorithm forecast those? The short answer is no. And this is why:
1. Measuring promo impact as % vs Baseline may be misleading
How does software measure promo impact? Most of the time it would just compare actual sales to the baseline. % difference would be the promo impact that the machine will use to forecast future promotions. However, reality might be a bit more complex than that. Imagine you are forecasting a winter ice cream promotion based on a previous summer promotion. Your summer baseline was 100 units and you sold 200 units as the result of the promotion. The software calculates a 100% lift. Impressive right? Let’s apply that to your winter baseline of 15 units. Not that impressive anymore. A human can create a promo lift that might not be calculated by a particular formula but nevertheless is reasonable.
2. Product life cycle trends can throw machines off
Most products have a sustain period after the launch. This is the period of the product life cycle right after the initial launch when sales gradually decline until they level off and start exhibiting seasonality. Software is not very efficient at forecasting this trend. It usually either underestimates future sales by applying the negative trend indefinitely or overestimates by applying seasonality to sales that are still elevated after the launch. A human can apply common sense to determine when the sustain period ends and normal seasonality starts.
3. Disentangling components of promo impact via algorithms may result in lower accuracy
Every new product promotion is unique with its own mix of marketing support, pricing and product strategy. Imagine you are forecasting a promotion based on two historical promotions. One historical promotion had a 10% lift and the other a 100% lift. An algorithm would use the average 60% lift for the forecast. In most cases this would be inaccurate. A human can make a judgement call on what particular factor differentiated the two historical promotions and choose the most similar one as a proxy for the forecast. I know what you’re going to say, “But we can teach the machine to recognize different kinds of promotions!” This brings me to my next point…
4. Teaching machines may be more work than doing it yourself
When a human analyses historical promotions he/she can look at different files, process different formats and even ask for other human opinions. A machine, however, demands to be spoon-fed data in a consistent format from one source. Creating a variable for every marketing strategy for every historical promotion can be very time-consuming.
5. Are you working on a forecast or on a model?
A statistician will say that all of the above issues may be resolved within a model by overriding product life cycle trend, flagging different kinds of promotions, using proxy seasonality, etc. This is true. The caveat is that these processes require a lot of human hours. Human hours dedicated to perfecting the machine, rather than perfecting the forecast. Anyone who has worked with models knows that statistical significance does not always go hand in hand with common sense. Overrides and interventions increase the risk of over-specification of the model. This means that model results stop making sense because there is not enough data to support all the additional variables.
6. Humans can think outside the box while machines are the box
Forecasting new products that have no data is solely reliable on proxy selection. Imagine you are forecasting a new LTO flavor of ice cream – dark chocolate. You have history on two LTOs – coffee and caramel chocolate ice cream. Which flavor will you choose as the proxy? A human will consider a plethora of factors. Which of the 2 historical flavors was more similar in taste? Is the bitterness or chocolatey-ness driving the sales? Is there any consumer research on the two flavors? Which of the promotions had similar marketing support? Was the creative more appealing for one of the historical flavours? All these questions belong to the qualitative, not quantitative realm. Hence, the software will not be helpful.
So what is the right balance between human and machine in forecasting? The answer is obvious – leave the quantitative tasks to the machines and qualitative to humans. The problem is that there is a fine line between the two in the realm of forecasting. The best term for a forecast in my mind is ‘a scientific guess’. We don’t want to leave the guessing part to the machines as it relies on intuition – an inherently human feature. It is worthwhile investing time in the exercise of classifying forecasts into ones that are generic enough to be done by machines and the ones that require a human touch. Machine and human can even efficiently cooperate within one forecast. For line extensions, software can predict the baseline level and even the promotional impact for the total line. Human can decide what proxy to use for the new flavor.
New product Forecasting Software Not Worth The Price
The good news is that software can effectively and efficiently forecast products with lots of history and homogeneous promotions. And they’re not expensive and are very easy to operate. There are even add-ons in Excel that can create baselines for hundreds of SKUs at different levels – provided you have enough data. It is the software that claims to forecast new products that can get pricey while providing dubious value add.
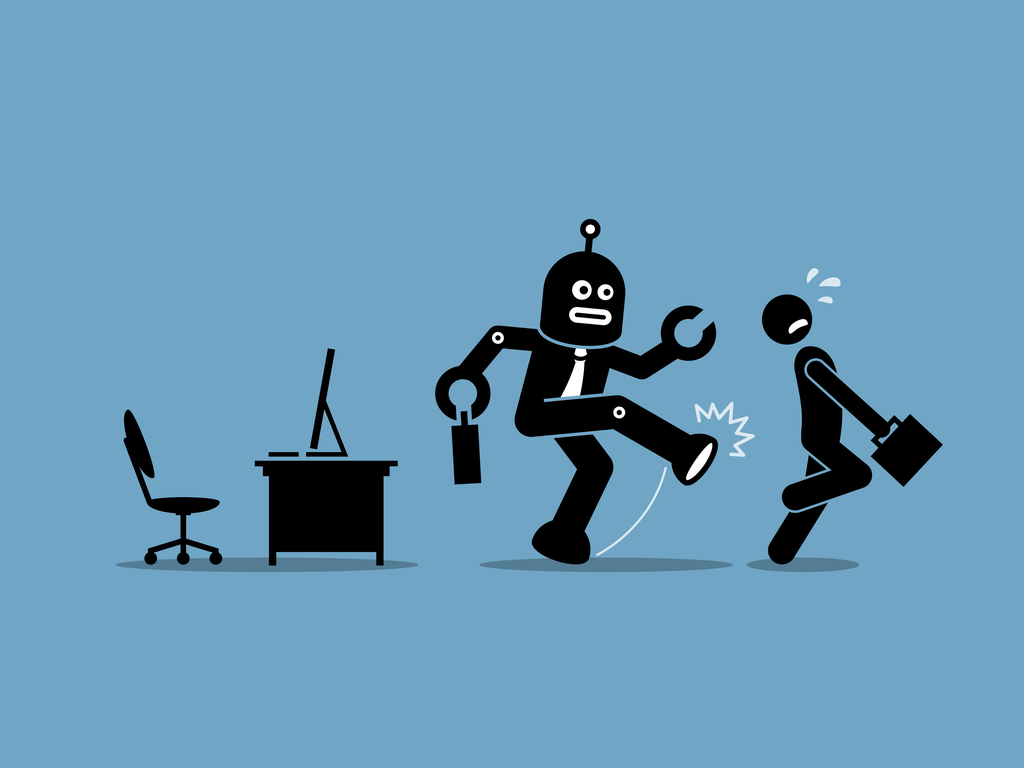
Demand planners needn’t fear being replaced by machines – only humans can provide valuable qualitative judgement.
Reconciling Quantitative & Qualitative Is Key In new Product Forecasting
Automation can release human time that can be spent on increasing forecast accuracy on the products that are the most difficult to forecast. There is a lot of room for improvement in the realm of forecasting. Average error for new food is around 50%. This equates to lost profits and excess inventory. One third of global food production is wasted, a problem that software alone will not resolve. Perfecting qualitative methods of forecasting is as important as quantitative, and even more important, is developing methods for reconciling the two. There are numerous consumer research specialists in the retail industry selling their qualitative expertise on new product appeal. Developing a system that converts such consumer research into real numbers that can be used for forecasting can significantly improve forecast error.