For many companies and demand planners, predictive analytics seems revolutionary. To others, it is just a more advanced version of normal demand planning. The concepts of behavior predictions can be traced back to the year of 1689 and many of the new machine learning algorithms like neural networks and ARIMA have been around for decades.
Can we really call them new and revolutionary? No, but they are replacing the tried and true methods demand planners have relied on. And we should know how predictive analytics differs from traditional forecasting, and what value it brings.
Even though these are concepts from over 40 years ago, we are seeing a renaissance in demand planning brought on by more data, technology advancements, and more consumer-driven markets. This is what is causing the current evolution from traditional time series forecasting to an approach that is more about understanding consumers.
Whereas traditional forecasting is all about the numbers and using level and trend and seasonality observations to predict outcomes, predictive analytics is more about consumer behavior and may use explanatory variables to predict outcomes. But this is just the tip of the iceberg. See table 1 for the many differences between traditional and revolutionary forecasting.
Table 1 | Traditional vs. Revolutionary Approach
Traditional | Revolutionary |
Demand Planning Forecasting Requirements based Limited demand factors Forecasts sales Tells you what to order Used when relationship between variables is strong Provides output to one specific question (what is demand) | Predictive Analytics Opportunity oriented Limitless factors Predicts drivers Tells us why the consumer buys Can be used to find relationships between unknown variables Provides multiple insights and solutions for the whole business |
Traditional demand planning primarily uses time-series and only a few demand factors. Even causal modeling for the most part consists of simple ratios and/or a single dependent variable that is used to extrapolate out.
Advanced predictive analytics uses a combination of models and techniques (which can include machine learning algorithms) to evaluate larger data sets with many more attributes, and can consider limitless amounts of fundamental factors at once.
Traditional demand planning is somewhat limited in its approaches and inputs, employing a single hierarchy and a single best fit model to forecast the product at hand.
With advanced predictive analytics we can predict sales of items and use similar algorithms and approaches to predict pricing, market share, weather, conversion rates, advertising, in-store merchandising, and a host of other variables at a multitude of dynamic hierarchies.
Traditional demand planning has been used historically for a reliable single point signal to produce or purchase products and manage inventory levels by providing a sales estimate for items.
Predictive Analytics Is A Business-Wide Activity
Advanced predictive analytics is being adopted by not only supply chains but also by marketing for promotional planning, and by sales for targeting customers likely to buy, and even for the purposes of customer retention and product management for portfolio optimization. It is accomplishing more by exposing more insights on why demand may occur and providing what if capabilities and drivers that may be used to influence demand. In contrast, Traditional demand planning typically works to a requirements-based model, answering clearly defined business questions like what will happen or what to order.
Advanced predictive analytics is revolutionary because it explores answers to ill-formed or even nonexistent questions.
A New Generation Of Data Junkies is Changing Forecasting Forever
Traditional demand planners have taken a strategic approach to managing forecasts and data (with varying degrees of success) for decades. They know how to acquire and house all relevant information and then build models to anticipate future results. Traditional information managers have historically taken a deductive approach by looking at their sales history, going through several rounds of collaboration and judgmental inputs, and working toward achieving one version of the truth.
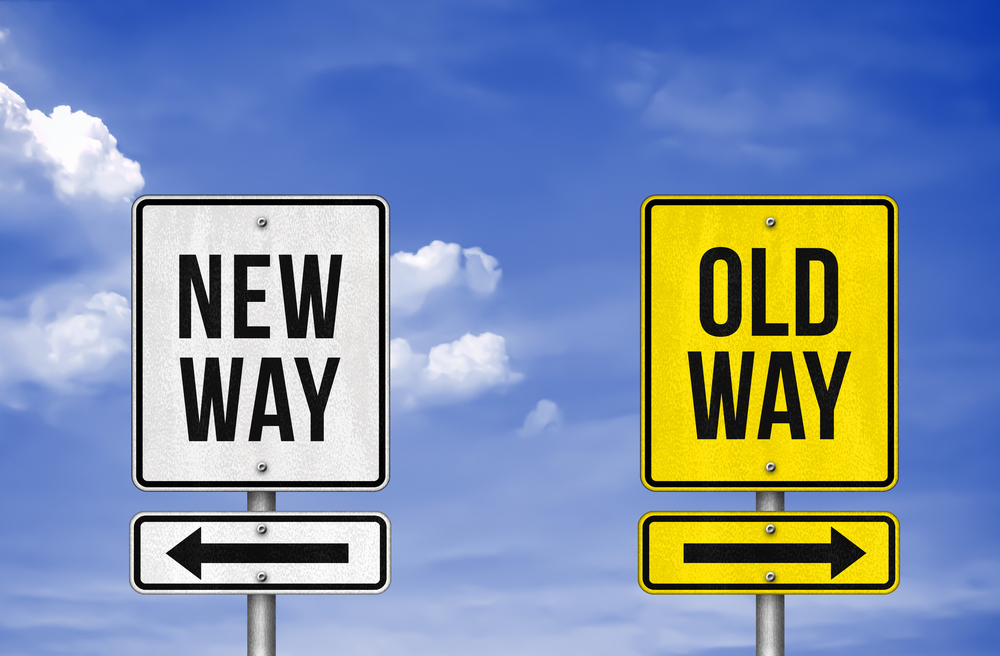
Given the availability of predictive analytics tools there is no excuse for not benefiting from the holistic business insight they provide.
Avant-garde big data professionals understand the corporate strategy or a concrete business question as the starting point; however, they look at it with a bottom-up approach. They believe that the innovation is in the data itself and that exploring this will lead to new answers, new drivers of their business, and new business strategies. Inside these innovative demand planning functions, a revolution is brewing, and a new generation of radical data junkies coming up through the ranks looks at information, forecasting, and demand-driven networks differently.
Inside these innovative demand planning functions, a revolution is brewing
Clearly, many are still stuck in the past. Excel as technology and Base-Lift models continue to be widely used for forecasting due to historical perception of sustainability challenges with predictive analytics. Techniques have not changed, or companies are reluctant to change, and thus operate in the same way they have been for years. They remain in their comfort zone because they are fearful, and they’re failing to progress to the next level and realize the potential of predictive analytics.
Eric will reveal how to update your S&OP process to incorporate predictive analytics to adapt to the changing retail landscape at IBF’s Business Planning, Forecasting & S&OP Conferences in Orlando (Oct 20-23)and Amsterdam (Nov 20-22). Join Eric and a host of forecasting, planning and analytics leaders for unparalleled learning and networking.