Along with the 14th of March, today should be our field’s public holiday. Why? Because today we celebrate one of the greatest forecasters and prognosticators in history, Mr. Punxsutawney Phil, our field’s hairiest analyst who provides his annual forecast on one of America’s most quirky holidays. This is unquestionably February 2nd and today is once again Groundhog Day.
His methods and results might be questionable but, us being in the field of business forecasting and demand planning, we should give him respect. He only comes out once a year and to make a prediction – it really doesn’t matter if he is right or not, and people throw him a party and celebrate what he says regardless. If only we were treated with the same respect at our companies.
For those living in a groundhog hole or live outside of our borders and wonder what these celebrations are about (I don’t blame you), every year we wait anxiously for the forecast of a famous groundhog from the little town of Punxsutawney, Pennsylvania. Since 1887, legend has it that if the groundhog named Punxsutawney Phil sees his shadow on February 2nd, six more weeks of winter weather lay ahead; no shadow indicates an early spring. Contrary to popular belief, Phil doesn’t actually have to see his shadow; he just has to cast one to make his wintery prophecy.
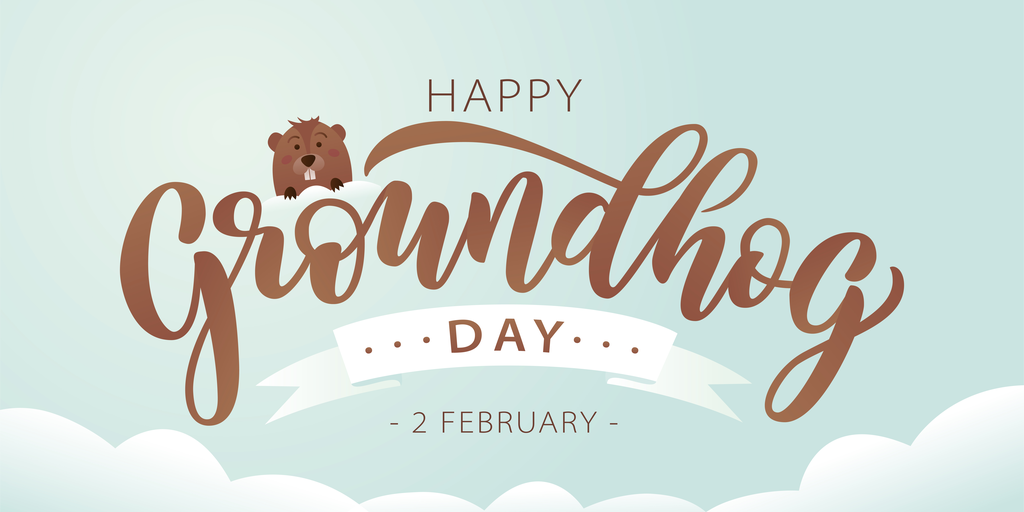
The new holiday for forecasting professionals? IBF wishes you a Happy Groundhog Day.
Phil Prefers a Random Walk Model
If we think about this, what Phil is doing is pretty much a naïve forecast. A naïve model is a model in which minimum amounts of effort and manipulation of data are used to prepare a forecast. The commonly used example is a random walk which uses the current or prior value as a forecast of the next period.
What Phil is doing in his forecast is taking the current period, February 2nd, and using that to forecast the next period or next six weeks. It is easy to make the mistake at looking at his “shadow model” as more of a causal model where the model is based on a cause-and-effect relationship where the independent variable of his shadow is correlated to the length of winter. In actuality he is relying more on time series data and simply doing a random walk using current observations to predict the next period.
How Accurate Is Phil?
In 2014, Phil was right on the money. After he saw his shadow, the country endured the 37th coldest February on record (1.6°F below the 20th century average) and the 43rd coldest March (1.0°F below the 20th century average). Compared with his other predictions, however, it seems that Phil might have just got lucky.
According to the Groundhog Club’s records, Punxsutawney Phil has predicted 108 forecasts of more winter and 18 early springs. If we look at the actuals for those same periods the data shows that 49 times Phil’s six-week prognostications were correct. Looking at the total his average Mean Absolute Percentage Error (MAPE) is just over 61 percent and has been correct about 39 percent of the time.
It also appears that this groundhog naïve or random walk prediction model is slightly better when he doesn’t see his shadow. Every time Phil predicted a short winter, he was much more likely to be right. Of the 18 times that he didn’t see his shadow and predicted an early spring, he got it right 8 times – that is a 44 percent accuracy rate.
Phil Should Consider a Seasonal Model
It would appear that, when looking at the forecast accuracy, Phil is committing one of the cardinal sins of forecasting. He may be selecting a model solely to fit history or, in this case, a naïve forecast based on fit to most recent history and ignoring other data or external factors.
Looking at the actuals, we have had 67 early springs and 59 late thaws and extended winters. I believe one of the key factors that Punxsutawney Phil may be missing is seasonality. Taking this into account, if he still wants to utilize a naïve forecast, it would serve him better to consider a seasonal random walk model that would take the prior period from the prior year instead of the current or last period.
An example of a seasonal random walk would be to use the actual from a year ago as the forecast for the next six weeks. Thus, Phil would have been correct a little over 53 percent of the time. And considering the contiguous United States just experienced its 18th consecutive year with an above-average annual temperature, Phil may be wise to use the seasonal random walk compared to the random walk model and more often predict an early spring.
Phil’s MAPE is Poor – Is Forecast Bias To Blame?
Another fatal flaw that may be impacting Phil’s predictions is an overly complex and politicized forecasting process. Politics and bias can be the most damaging influence to a forecast and worse than poor forecast accuracy. Looking at Phil’s results his Mean Percentage Error (MPE) for the 126 years is 33 percent and in the past 20 years Phil has over-forecasted (projected longer winters with spring actually coming earlier) over 40 percent of the time.
Unfortunately, Phil is not alone and any forecasting process can be degraded in various places by the biases and personal agendas of participants. It seems the more elaborate the process, with more human touch points, the more opportunity exists for these biases to pollute what should be an objective and unbiased process. That said, can we really believe a rodent when it is being manipulated by multiple human touch points and a third party is interpreting his forecast?
Do We Have an Unrealistic Expectation of Forecast Accuracy?
I refuse to repeat the mantra of “the forecast is always wrong” but, forecast accuracy is ultimately limited by the nature of the behavior of what we are trying to forecast. There is inherent variability in the data and many times inherent flaws in the process. With Phil, logic would conclude that we have roughly a little bit better than a 50 percent probability of forecasting an early spring. Why would we expect him to do better than that with the tools he is provided? Consider this same statement when judging the forecast accuracy of your team or your own forecasting ability.
If the behavior is wild and erratic (referring to the data, not the groundhog) with no structure or stability, then we have no hope of using it to generate a reasonable forecast, no matter how much time, money, and ceremony goes into it. The most sophisticated methods cannot forecast unforecastable behavior, and we have to learn to live with that reality.
Should We Depend On A Marmot As A Genuine Forecasting Method ?
I think the ultimate lesson here is not that Phil is wrong, but he falls prey to many of the same forecast problems that we face every day. What we can learn from Phil is how not to fall into those traps ourselves and work on taking bias out, finding the right models and proper use of fitting, understanding variability and probabilities, and using error to improve instead of judge. Finally, the greatest lesson Phil teaches us is that no matter how bad your forecasts are, there is still hope to become a celebrity and have your company celebrate you for what you do.